Part of what makes The Tie Terminal the leading institutional crypto data platform is our best-in-class Social Media & Sentiment data. In crypto markets, Social Media conversation can have outsized impact on an asset's performance relative to traditional markets - therefore, it is imperative that investors have access to a wealth of data around not only raw conversation volumes, but also quantified sentiment scoring for hundreds of projects.
The Tie
Social Media & Sentiment Data Deep Dive
This article aims to deliver a comprehensive understanding of The Tie's Social Media & Sentiment Data, detailing our collection, cleaning, and sentiment scoring processes.
Ensuring Cleanliness & Actionability of our Data
Our research indicates that more than 90% of conversations on Twitter/X about cryptocurrencies come from potentially inaccurate, fraudulent, or manipulative users.
With such a high volume of inauthentic activity, we leverage patented technology to separate signal from noise and detect/eliminate inorganic Twitter conversations in the metrics we deliver to The Tie Terminal and our Social Media API. Through account filtration, manipulation detection, and account accuracy technology, we ensure that the data we provide to our institutional users is both clean and actionable.
Our engine looks at how an individual user's tweets correspond to the price action of the assets they're tweeting about, which enables our data to exclude users whose tweets are consistently poor predictors of performance. Further, we look at dispersion, or the percentage of tweets about an asset coming from unique accounts. When dispersion is low (a small percentage of tweets are coming from unique users), it is often the case that a concentrated group of users is trying to manipulate conversations to influence price movement. Our engine considers these cases and excludes activity it deems to be manipulative.
Lastly, to ensure our data reflects only authentic activity, we assess key account-level metrics like account age, followers to following ratio, and posting history. When an account is posting extensively about a singular asset, or sharing a high volume of extremely positive (or negative) tweets in bulk, our engine flags this activity as inauthentic and excludes these flagged tweets/accounts from our analysis.
Through this multifaceted process of cleaning and vetting, we ultimately eliminate more than 90% of total crypto-specific Twitter activity before we quantify Sentiment & Conversation Volume metrics to The Tie Terminal and our Social Media API.
Sentiment, Defined
Sentiment is a quantified (relative) representation of investors' future intentions. For our purposes, it matters less that John Doe is happy with Bitcoin's price today - what matters is whether they are bullish or bearish on the future of the asset. Further, it's crucial that collectively, investors' sentiment towards an individual asset in a certain time period is more positive than over a previous period for sentiment levels to be comparable across assets.
The Tie's Sentiment Scoring - a Four-Step Process
Our process leverages X/Twitter's firehose - a real-time feed of more than a billion tweets per week. This allows us to cast a wide net across the X/Twittersphere and capture all relevant activity.
The figure below reflects an overview of our sentiment scoring process:
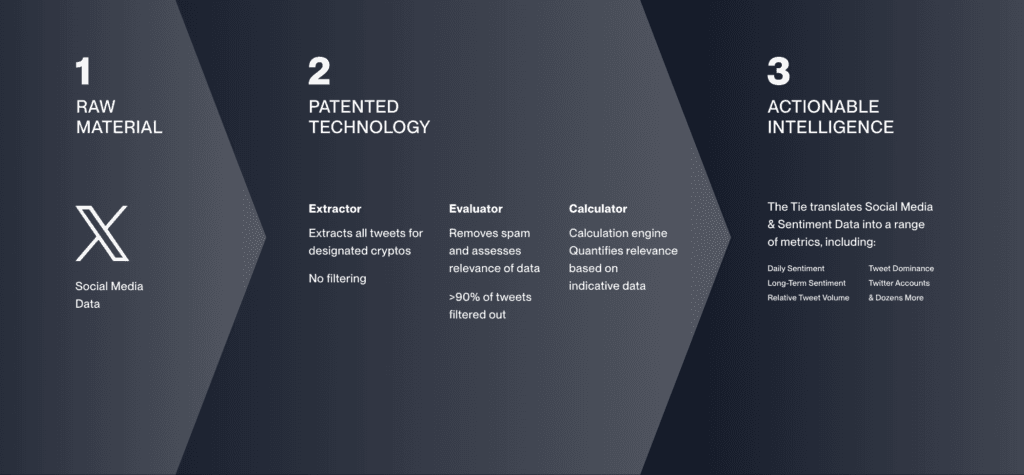
Step 1 - Ensure Relevance
We begin by assessing the relevance of a tweet to a corresponding crypto asset, and extracting/bucketing all tweets related to the same topic. This can be more complex than it may seem - there are countless instances where a cryptocurrency's name or ticker overlaps with an entirely unrelated topic. For example, Cardano's ticker ADA must be considered distinct from tweets about the Americans with Disabilities Act (ADA). Additionally, it is crucial that our coverage of Ripple (XRP) does not consider the more generic term 'ripple,' where someone could reasonably tweet that Apple's declining earnings have a ripple effect across the NASDAQ more broadly. Likewise, coverage of the NEAR token must be analyzed separately from tweets that contain the word 'near.' One final illustrative example - our coverage of Avalanche (AVAX) must exclude tweets related not only to the winter weather event, but also the NHL franchise, the Colorado Avalanche.
To ensure our coverage's relevance and connection to the crypto market, we developed topic models that assess every tweet's context and every user's historical patterns. While this process is largely automated, it also requires human supervision and updating to ensure the models are sufficiently refined to avoid associating irrelevant tweets. This patented process removes more than 99% of irrelevant activity.
Step 2 - Account Filtration
With tweets extracted and associated with an asset, we then apply our Account Filtration, a process discussed in more detail in the section above, which removes manipulative and fraudulent post. In this second step, more than 90% of tweets are filtered out.
Step 3 - Scoring Sentiment
This step analyzes and scores each word in a tweet, via machine learning that leverages a dictionary of more than 100,000 unique terms to determine positivity or negativity. By assessing each word in the tweet, the tweet receives a composite score. An example of this process is illustrated below:
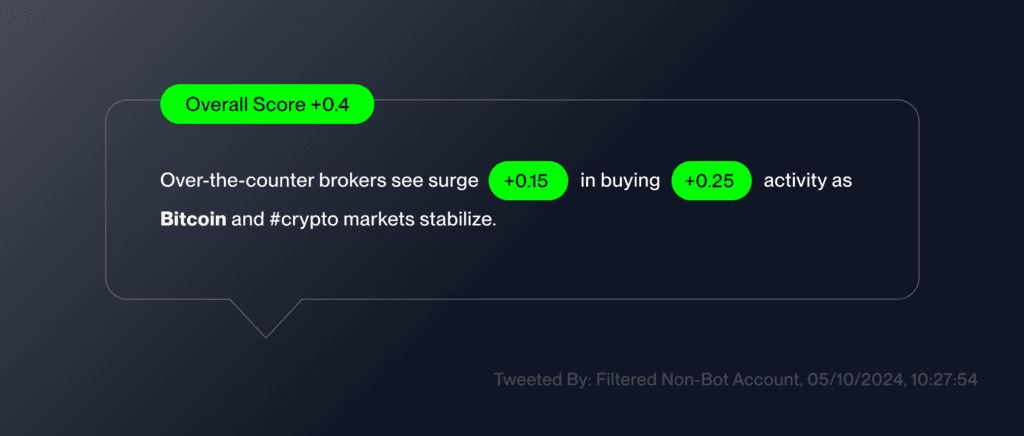
In this example, "surge" and "buying" are both scored positively, and the tweet receives an overall score of +0.4.
Step 4 - Aggregation & Metric Creation
In our final step, we aggregate individual scored tweets into quantified and actionable metrics for traders and investors. By normalizing the data and comparing sentiment over different lookback periods, we can identify when an asset's conversations are becoming increasingly positive or negative on different time scales.
With this step, it's crucial to note that we do not compare conversations between two assets on a like-for-like basis - certain cryptocurrencies have, on average, more positive conversations than others. For example, DOGE has a large community that supports the asset on Twitter, and on average, conversations about DOGE are much more positive than those for Bitcoin.
Therefore, our Sentiment scores compare conversations around an asset over one period versus conversations on the same asset over another period. For example, to calculate a Daily Sentiment Score, we look at a statistical score (similar to a z-score) of how positive Bitcoin's conversations are today compared to the last 20 days. Once we normalize the data, if we find that Bitcoin sentiment is two standard deviations more positive today relative to the last 20 days, there would be an increase in Bitcoin's Daily Sentiment Score metric. The final score is normalized between 0 and 100, 0 corresponding to the most negative sentiment score, 50 to neutral sentiment, and 100 to the most positive sentiment score.
All scores are calculated every minute to provide granular live and historical sentiment data on the 250+ tokens we support.
Conclusion
Social Media and Quantified Sentiment data are uniquely insightful and actionable in crypto markets, where narratives and momentum around a project can inspire retail investors and institutions alike to consider investing. Through The Tie's proprietary technology for tagging and scoring, we are positioned to deliver institutions the cleanest, most accurate, and therefore most actionable social media analytics in crypto. Whether through our flagship product, The Tie Terminal, or through our Social Media API, institutions can take advantage of this crucial alternative dataset to detect market trends, identify narratives, built market intuition, or attempt to predict price movements.
It is important to note that in our Social Media API, all Tweet data collected is point-in-time, enabling thorough backtesting and validation. Find more details about our Social Media API in our Docs.
To learn more about The Tie Terminal and our Social Media data coverage, schedule a demo with our team here.
Sign up to receive an email when we release a new post
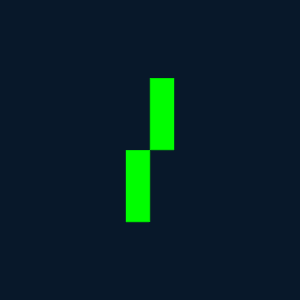